BSTARS 2018
2018 Berkeley Statistics Annual Research Symposium (BSTARS)
The Berkeley Statistics Annual Research Symposium (BSTARS) surveys the latest research developments in the department, with an emphasis on possible applications to statistical problems encountered in industry. The conference consists of keynote lectures given by faculty members, talks by PhD students about their thesis work, and presentations of industrial research by alliance members. The day-long symposium gives our graduate students, faculty, and industry partners an opportunity to connect, discuss research, and review the newest development happening on-campus and in the field of statistics.
Schedule
BSTARS 2018 will be March 12th, 1:30pm-8:30pm, at The Alumni House, UC Berkeley.
1:30-2:00 | Arrival, coffee, and pastries |
2:00-2:10 | Welcome and opening remarks
|
2:10-3:15 | Thunder Talks |
3:15-3:30 | Break |
3:30-4:55 | Thunder Talks 2 |
4:55-6:00 | Poster Sessions |
6:00-6:45 | Keynote by Professor Fernando Perez |
6:45-8:30 | Dinner |
Keynote Speaker- Fernando Pérez
Building an open platform for research and education in data science with Project Jupyter
Project Jupyter, evolved from the IPython environment, provides a platform for interactive computing that is widely used today in research, education, journalism and industry. The core premise of the Jupyter architecture is to design tools around the experience of interactive computing. It provides an environment, protocol, file format and libraries optimized for the computational process when there is a human in the loop, in a live iteration with ideas and data assisted by the computer.
I will discuss both how the architecture of Jupyter supports a variety of workflows that are central to the processes of research and education. In particular, Jupyter supports reproducible scientific research and the communication of data-intensive narratives both within the scholarly community and with broader audiences. By providing tools that can benefit research scientists as well as media practitioners and journalists, we hope to contribute to a more informed debate in society in domains where data, computation and science are key.
Professor Pérez is an Assistant Professor in Statistics as well as a Faculty Scientist in the Department of Data Science and Technology at Lawrence Berkeley National Laboratory. His research focuses on creating tools for modern computational research and data science across domain disciplines, with an emphasis on high-level languages, interactive and literate computing, and reproducible research. He created IPython while a graduate student in 2001 and co-founded its successor, Project Jupyter. The Jupyter team collaborates openly to create the next generation of tools for human-driven computational exploration, data analysis, scientific insight and education. He is a National Academy of Science Kavli Frontiers of Science Fellow and a Senior Fellow and founding co-investigator of the Berkeley Institute for Data Science. He is a co-founder of the NumFOCUS Foundation, and a member of the Python Software Foundation. He is the recipient of the 2012 Award for the Advancement of Free Software from the Free Software Foundation.
Thunder Talks by Industry Alliance Program Members
Citadel Presenter: Tao Shi, Quantitative Researcher & Benedikt Lotter, Quantitative Researcher
State Street Presenter: John S. Arabadjis, Managing Director, Head of GX Labs
TGS Management Group Presenter: Phil Naecker, CTO
Two Sigma: Xufei Wang, Quantitative Researcher
Uber Presenter: Sreeta Gorripaty, Data Scientist
UniData Presenter: Maple Xu, Co-Founder
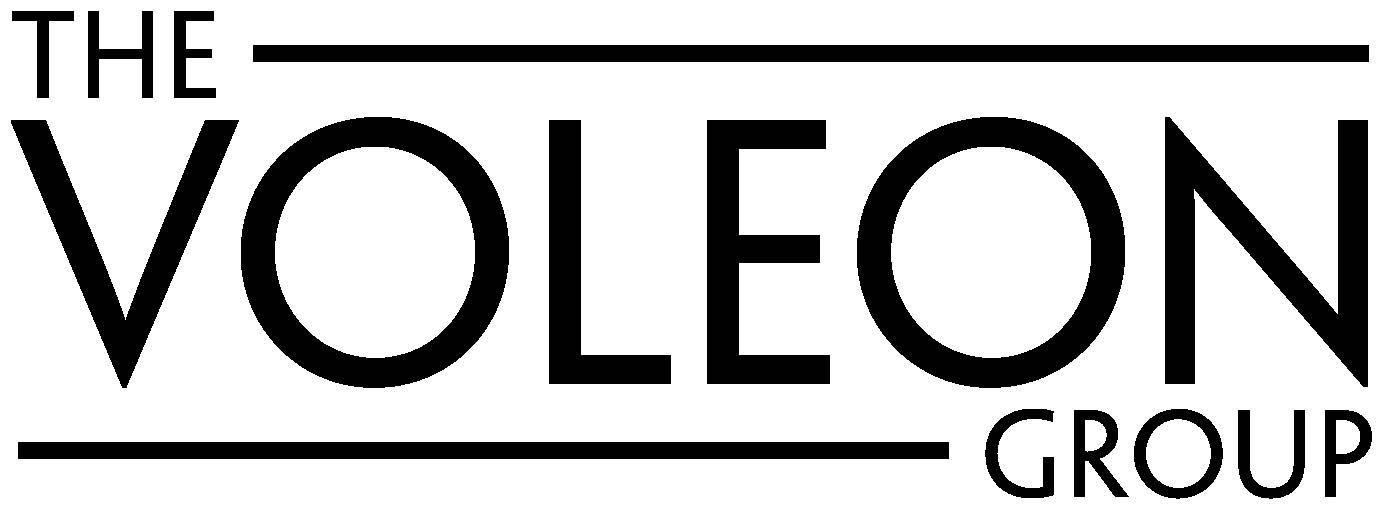
Voleon Presenter: Lauren Hannah
Thunder Talks and Poster Presentations by PhD Students
Eli Ben-Michael, Statistics Department
It's always about selection: The role of the propensity score in synthetic control matching
The synthetic control method is a popular approach for estimating the effect of a treatment, typically for a single treated unit and multiple control units, when (typically many) pre-treatment outcomes are observed for all units. The literature on synthetic controls, however, has largely emphasized modeling the outcome process, rather than assumptions on selection into treatment. We address this gap in the literature by showing that the synthetic controls method has a dual view as an inverse propensity score weighting estimator with a regularized propensity score. We also show that this estimator is (approximately) doubly robust whenever an (approximately) adequate synthetic control can be constructed: the method is consistent under misspecification of either the selection process or the outcome model. We combine these theoretical results with extensive simulations to show that the validity of the synthetic control method is sensitive to changes in the selection process and the outcome model. Finally, we use this dual perspective to build intuition for non-standard settings, such as estimating effects for multiple outcomes, and suggest modifications to improve the performance of synthetic control methods in practice.
Kevin Kiane Jacques Benac & Nima Hejazi, Biostatistics Department
Efficient Estimation of Survival Prognosis Under Immortal Time Bias
We consider the problem of efficiently estimating survival prognosis under a data structure complicated by the presence of immortal time bias. Where the fundamental concern of survival analysis is the estimation of time-to-event outcomes, possibly subject to left or right censoring, the matter of efficient estimation under a bias induced by time-dependent risks presents a novel challenge that has received surprisingly meager attention in the literature. The present problem examines data on observations potentially subject to multiple survival processes, where individuals in a cohort are followed starting with an indexing event (a risk-increasing survival process) until death, with a subset of individuals shifting from the baseline risk profile to a secondary risk profile prior to death. We compare both parametric and nonparametric estimators of survival, including variations of the Cox proportional hazards model and the Kaplan-Meier estimator, evaluating the efficiency of each in the estimation of the multiple survival processes that occur under this data-generating process. We illustrate the utility of employing the nonparametric estimator that we propose via simulation studies; moreover, we motivate our investigation with examples of inappropriate applications of these estimators in the medical literature, and, time permitting, an analysis of observational medical data that exemplifies the corrections provided by our estimator of choice.
Arturo Fernandez, Statistics Department
A Statistical Framework for Modeling Tropical Cyclone Genesis
Tropical cyclones (TCs) are important extreme weather phenomena that have significant negative impact on humans, infrastructure, and society. Multidecadal simulations from high resolution regional and global climate models are now being used to better understand how TC statistics change due to anthropogenic climate change. Aspects of tropical cyclones such as their genesis, evolution, intensification, and dissipation over land are important and challenging problems in climate science. Although various studies have been conducted on the climatology of tropical cyclone genesis (TCG), their analyses are often limited in that they focus on basin-specific models, discard high resolution data in favor of aggregate measures, or bias their investigation towards variables and metrics that are motivated by mathematical physics.
This study investigates environmental conditions associated with TCG by testing how accurately a statistical model can predict TCG in the Community Atmospheric Model (CAM) Version 5.1. The defining feature of this study is the use of multi-variate high resolution data without any imposed criteria or physical constraints. TC trajectories in CAM5.1 are defined using the Toolkit for Extreme Climate Analysis (TECA) software [TECA: Petascale Pattern Recognition for Climate Science, Prabhat et al., Computer Analysis of Images and Patterns, 2015] and are based on standard criteria used by the community (thresholds on pressure, temperature, vorticity, etc.). L1-regularized logistic regression (L1LR) is applied to distinguish between TCG events and non-developing storms. In this study we define a developing storm event as a tropical depression that matures into a TC (Cat 0 through 5) and a non-developing storm event as that which does not. We assess our model on two sets of test data. First, when tested on data with no TC track association (no storm events) the model has near perfect accuracy. Secondly, when differentiating between developing and non-developing storm events, it predicts with high accuracy.
The model’s active variables are generally in agreement with current leading hypotheses on favorable conditions for TCG, such as cyclonic wind velocity patterns and local pressure minima. However, other variables such as a sea surface temperature, precipitation, and vertical wind shear are seen to have marginal influence. We note that this is not contradictory with existing physical understanding of the mechanisms of TCG because the goal of this model is to achieve high predictive accuracy with no imposed physical constraints. Hence the model discovers the variables and spatial patterns that maximize predictive accuracy, irrespective of their role in the physics of TCG. Therefore it is also reasonable to expect that the model may only use the variables that are most influential in predicting TCG and not necessarily all the variables associated with the physics of TCG. Furthermore, our model’s predictions of the climatology of TCG exceed the predictive ability of instantaneous versions of other physically and climatologically-motivated indices such as the Genesis Potential Index (GPI), Ventilation Index, and Entropy Excess.
Johnny Hong, Statistics Department
A Spectral Approach to Incorporate Phylogenetic Signals
In ecological modeling, such as species occupancy models, Pagel's lambda is a popular approach to integrate phylogenetic signals into the analysis. We develop an extension of Pagel's lambda that is based on spectrum modification of the expected correlation matrix from a Brownian motion phylogeny. Our proposed framework not only provides greater modeling flexibility, but also delineates how various components of phylogenetic signals are reflected in species traits.
Steve Howard, Statistics Department
Nonasymptotic sequential estimation with uniform Chernoff bounds
The rise of online A/B testing has created new needs in sequential experimentation which are not well-served by existing methods. We describe an extension of Chernoff's method to uniform concentration bounds, with connections to classical sequential analysis, and discuss how to build on this idea to devise useful nonasymptotic methods for sequential experimentation in practice. We give example applications in ATE estimation under the potential outcomes model and in matrix estimation.
Koulik Khamaru, Statistics Department
Convergence guarantees for a class of non-convex and non-smooth optimization problems
We consider the problem of nding critical points of functions that are non-convex and non-smooth. Studying a fairly broad class of such problems, we analyze the behavior of three gradient-based methods (gradient descent, proximal updates, and Frank-Wolfe algorithm). For each of these methods, we establish rates of convergence for general problems, and also exhibit faster rates for sub-analytic functions. We also show that our algorithms can escape strict saddle points for a large class of non-smooth functions, thereby generalizing know results for smooth functions. Finally, as an application of our theory, we obtain a simplication of the popular CCCP algorithm, used for optimizing functions that can be written as a dierence of two convex functions. We show that our simplied algorithm retains all the convergence properties of CCCP, along with a signicantly lower cost per iteration. We illustrate our methods and theory via application to the problems of best subset selection, robust estimation, and mixture density estimation.
Karl Kumbier, Statistics Department
Iterative Random Forests to discover predictive and stable high-order interactions
Individual genomic assays measure elements that interact in vivo as components of larger molecular machines. Understanding how these high-order interactions drive gene expression presents a substantial statistical challenge. Building on Random Forests (RF), Random Intersection Trees (RITs), and through extensive, biologically inspired simulations, we developed the iterative Random Forest algorithm (iRF). iRF trains a feature-weighted ensemble of decision trees to detect stable, high-order interactions with same order of computational cost as RF. We demonstrate the utility of iRF for high-order interaction discovery in two prediction problems: enhancer activity in the early Drosophila embryo and alternative splicing of primary transcripts in human derived cell lines. By decoupling the order of interactions from the computational cost of identification, iRF opens new avenues of inquiry into the molecular mechanisms underlying genome biology.
Lihua Lei, Statistics Department
AdaPT: An interactive procedure for multiple testing with side information
We consider the problem of multiple hypothesis testing with generic side information: for each hypothesis Hi we observe both a p-value pi and some predictor xi encoding contextual information about the hypothesis. For large-scale problems, adaptively focusing power on the more promising hypotheses (those more likely to yield discoveries) can lead to much more powerful multiple testing procedures. We propose a general iterative framework for this problem, called the Adaptivep-value Thresholding (AdaPT) procedure, which adaptively estimates a Bayes-optimal p-value rejection threshold and controls the false discovery rate (FDR) in finite samples. At each iteration of the procedure, the analyst proposes a rejection threshold and observes partially censored p-values, estimates the false discovery proportion (FDP) below the threshold, and proposes another threshold, until the estimated FDP is below α. Our procedure is adaptive in an unusually strong sense, permitting the analyst to use any statistical or machine learning method she chooses to estimate the optimal threshold, and to switch between different models at each iteration as information accrues. We demonstrate the favorable performance of AdaPT by comparing it to state-of-the-art methods in five real applications and two simulation studies.
Bryan Liu, Statistics Department
Measuring Cluster Stability for Bayesian Nonparametrics Using the Linear Bootstrap
Clustering procedures typically estimate which data points are clustered together, a quantity of primary importance in many analyses. Often used as a preliminary step for dimensionality reduction or to facilitate interpretation, finding robust and stable clusters is often crucial for appropriate for downstream analysis. In the present work, we consider Bayesian nonparametric (BNP) models, a particularly popular set of Bayesian models for clustering due to their flexibility. Because of its complexity, the Bayesian posterior often cannot be computed exactly, and approximations must be employed. Mean-field variational Bayes forms a posterior approximation by solving an optimization problem and is widely used due to its speed. An exact BNP posterior might vary dramatically when presented with different data. As such, stability and robustness of the clustering should be assessed.
A popular mean to assess stability is to apply the bootstrap by resampling the data, and rerun the clustering for each simulated data set. The time cost is thus often very expensive, especially for the sort of exploratory analysis where clustering is typically used. We propose to use a fast and automatic approximation to the full bootstrap called the "linear bootstrap", which can be seen by local data perturbation. In this work, we demonstrate how to apply this idea to a data analysis pipeline, consisting of an MFVB approximation to a BNP clustering posterior of time course gene expression data. We show that using auto-differentiation tools, the necessary calculations can be done automatically, and that the linear bootstrap is a fast but approximate alternative to the bootstrap.
Ivana Malenica, Biostatistics Department
Robust Estimation of Causal Effects Based on Observing a Single Time Series
Causal inference from time-series data is a crucial problem in many fields. In particular, it allows tailoring interventions over time to evolving needs of a unit, painting a granular picture of the current status. In medicine, wealth of information available in time-series data hints at an exciting opportunity to explore the very definition of precision medicine- studies that focus on a single person. We present targeted maximum likelihood estimation (TMLE) of data-dependent and marginal causal effects based on observing a single time-series. A key feature of the estimation problem is that the statistical inference is based on asymptotics in time. We focus largely on the data-dependent causal effects that can be estimated in a double robust manner, therefore fully utilizing the sequential randomization. We propose a TMLE of a general class of averages of conditional causal parameters, and establish asymptotic consistency and normality results. Finally, we demonstrate our general framework for the data-adaptive setting with a number of examples and simulations, including a sequentially adaptive design that learns the optimal treatment rule for the unit over time.
Zvi Rosen, Mathematics Department
Geometry of the sample frequency spectrum and the perils of demographic Inference
The sample frequency spectrum (SFS), which describes the distribution of mutant alleles in a sample of DNA sequences, is a widely used summary statistic in population genetics. The expected SFS has a strong dependence on the historical population demography and this property is exploited by popular statistical methods to infer complex demographic histories from DNA sequence data. Most of these inference methods exhibit pathological behavior, however. Using tools from algebraic and convex geometry, we explain this behavior and characterize the set of all expected SFS.
Omid Shams Solari, Statistics Department
MuLe: A Power-Method For Robust CCA Problem
A new reformulation of the sparse Canonical Correlation Analysis, sCCA, problem is presented where the non-convex and therefore computationally non-tractable objective is reformulated as an optimization program of maximizing a convex objective over a convex set. Hence a more tractable solution. This drastically shrinks the search space which results in a signicantly faster algorithm. A rst order gradient method is then proposed for the program which has the best convergence properties when the objective and the feasible set are convex which is true n our case. We will also show that our method outperforms other methods both in quality of the canonical covariates and the computation cost in simulations and real datasets, some of which contain up to 1e6 covariates where leading existing methods fail to even handle.
Jake Soloff, Statistics Department
Identifying the Effect of Charter Schools via Matching
One source of contention in the debate on charter schools is the claim that they drain resources from the rest of the school district. We study the short-term impact of opening a charter school on district-level educational outcomes. Our identification strategy involves matching districts on demographic data prior to the introduction of a charter school. We consider the scope and limitations of this approach. This is joint work with Sören Künzel, Allen Tang, and Eric Munsing based on our submission to the 2017 Citadel Data Open Championship.
Sara Stoudt, Statistics Department
Clarifying the Identifiability Controversy in Species Distribution Modeling
We are interested in tracking plants and animals over time and space and understanding how their prevalence (proportion of area where they are found) and abundance (quantity) change. Collecting data is expensive, so it is important to know what data collection protocols we need to use in order to have enough information to estimate these quantities of interest. There has been controversy in the literature about the data quality resulting from certain protocols and the realism of certain model assumptions needed to estimate quantities of interest from this data. To clear up the controversy, we introduce different forms of identifiability from the econometrics literature and show why model misspecification can be especially dangerous when we have a weaker form of identifiability (parametric) instead of the strongest form (nonparametric).
Andre Wacshka, Statistics Department
A Cross-Validated Tuning Parameter Estimator for Decision Trees
The augmented tree-based method presented here is a procedure that uses cross-validated, variance-bias trade-off to choose the most refined level of stratification in order to minimize misclassification rates, by incorporating differential impacts for a false positive (FP) versus false negative (FN) rates. The new tree-based estimator is characterized by a tuning parameter α, which is a loss matrix composed of user-supplied weights (FP; FN). Our optimized CV method directly optimizes the weighted FP to FN ratio while capitalizing on the properties of cross-validation to limit the risk of overfitting. This yields an estimator that minimizes the cross-validated risk estimates.
Clinical applications of this approach suggest this method has great promise as a statistical tool for precision medicine.
Simon Walter, Statistics Department
On the modified outcome method for estimating heterogeneous treatment effects
We would like to estimate the expected treatment effect in a randomized experiment as a function of observed pre-treatment covariates; we explore the modified outcome method of Signorovitch (2007) for this purpose. In the simplest case, where the two equiprobable treatment assignments are indicated by $W_i$, the modified outcome method fits a regression model to: $Y_i^* = \frac{1}{2} (2W_i -1) Y_i$, a quantity equal in expectation to the individual treatment effect. We review seven results: (1) we give a new motivation for the modified outcome method; (2) we introduce notions of optimality for regression adjustment when using the modified outcome method to estimate the conditional average treatment effect (CATE); (3) we show that all optimal estimators of the CATE can be cast as modified outcome estimators with optimal regression adjustment; (4) we show that under minimal assumptions regression adjustment is asymptotically helpful even when the adjustment model is incorrect; (5) we show that when the true treatment effect is a linear function of the covariates then using the modified outcome method with the lasso converges to the CATE when certain regularity conditions are met and $\log p \ll n$; (6) we provide asymptotically consistent confidence intervals for parametric models of the treatment effect using Huber-White standard errors; (7) we show by way of simulation and application to real data that the modified outcome method with optimal regression adjustment is competitive with many existing approaches to estimate the CATE.
Yu Wang, Statistics Department
Individual prevalence: a new way to look into random forest
Iterative random forest model (iRF) is a powerful tool to identify high order feature interactions in the high dimensional data. However, it favors feature interactions that is important for the whole data. By introducing individual prevalence, we are able to examine the effect of feature interactions for every sample. By using individual prevalence, we identify low-dimensional clustered patterns in the enhancer data of Drosophila blastoderm as well as novel feature interactions.
Poster Presentations
The following graduate students and postdocs will also be presenting posters
PhD students: Stephanie DeGraff - Statistics Department, Suzanne Dufault - Biostatistics Program*, Jonathan Fischer- Statistics Department, & Aurelien Bibaut - Biostatistics Program*
Master's students: Yue You - Biostatistics Program*
*= One minute poster pitch