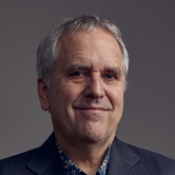
Michael Jordan
computer science, artificial intelligence, computational biology, statistics, machine learning, electrical engineering, applied statistics, optimization
I have interests that span the spectrum from theory to algorithms to applications. I'm most interested in problems that arise when working with non-traditional data types; examples I've worked with include document corpora, graphs, protein structures, phylogenies and multi-media signals. Working with these kinds of data types often leads one to work on problems of an unusually large scale, where classical methods can be infeasible on computational grounds. I've thus been interested in new computational methods for large-scale problems; specifically I've worked on the development of novel estimators using tools from constrained optimization theory and convex analysis. I'm also interested in the interface between probability theory and nonparametric statistics, particularly in the setting known as "nonparametric Bayes", where the prior distribution is a general stochastic process. Here ideas familiar in modern probability theory, such as the Chinese restaurant process and stick-breaking distributions, yield novel statistical models and novel inference procedures. These methods have numerous applications in areas such as statistical genetics, image processing and natural language processing. I'm quite interested in pursuing these applications, particularly in collaboration with biologists and computer scientists.