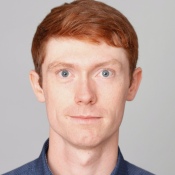
Alexander Strang
Stochastic Processes, Hierarchical Bayesian Inference, Random Graph Theory, Multi-Agent Training, Computational Biology, Solution Continuation, Optimization
Alexander Strang is an applied mathematician with a passion for teaching, mentoring, and leadership. He earned his Ph.D. in applied mathematics from Case Western Reserve University in 2020 and was a Kruskal Instructor in Statistics at the University of Chicago until 2023, where he won the Suzuki prize for outstanding postdoctoral work. His work is strongly interdisciplinary with roots in statistical physics, spectral graph theory, optimization, and mathematical biology. He has developed optimization and solution continuation algorithms for sparsity-promoting hierarchical Bayesian models, studied self-organization in evolving networks and populations, leveraged the spectral characterization of games to analyze decision problems, introduced moment closure bounds based on algebraic geometry, analyzed early warning signals in noisy dynamical systems, and applied of simplicial cohomology to stochastic processes near to equilibrium. He is interested in the interplay between structure and dynamics in complex networks.