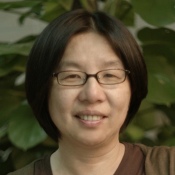
Bin Yu
statistical inference for high dimensional data and interdisciplinary research in neuroscience, remote sensing, and text summarization
I am The Class of 1936 Second Chair in the College of Letters and Science, and Chancellor's Distinguished Professor, Departments of Statistics and of Electrical Engineering & Computer Sciences, University of California at Berkeley.
My current research focuses on practice, algorithm, and theory of statistical machine learning and causal inference. My group is engaged in interdisciplinary research with scientists from genomics, neuroscience, and precision medicine. In order to augment empirical evidence for decision-making, we are investigating methods/algorithms (and associated statistical inference problems) such as dictionary learning, non-negative matrix factorization (NMF), EM and deep learning (CNNs and LSTMs), and heterogeneous effect estimation in randomized experiments (X-learner). Their recent algorithms include staNMF for unsupervised learning, iterative Random Forests (iRF) and signed iRF (s-iRF) for discovering predictive and stable high-order interactions in supervised learning, contextual decomposition (CD) and aggregated contextual decomposition (ACD) for interpretation of Deep Neural Networks (DNNs).
My past work covered research areas in empirical process theory, information theory (MDL), MCMC methods, signal processing, machine learning, high dimensional data inference (e.g. sparse modeling (boosting and lasso), and spectral clustering).
I am a member of the U.S. National Academy of Sciences and a fellow of the American Academy of Arts and Sciences. I was a Guggenheim Fellow in 2006, and the Tukey Memorial Lecturer of the Bernoulli Society in 2012. I was President of IMS (Institute of Mathematical Statistics) in 2013-2014 and the Rietz Lecturer of IMS in 2016. I received the E. L. Scott Award from COPSS (Committee of Presidents of Statistical Societies) in 2018, and was the Breiman Lecturer at NeurIPS 2019.